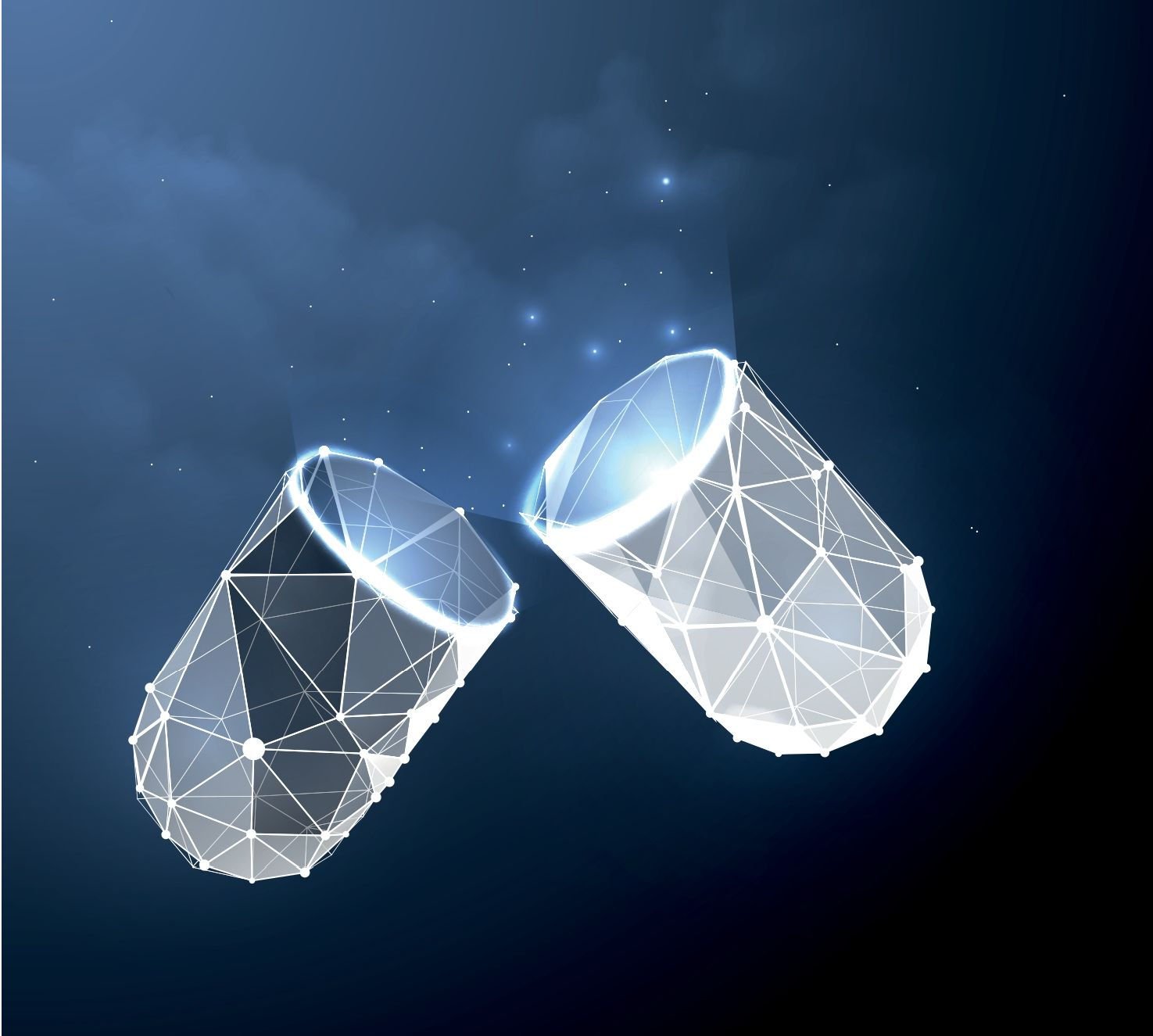
AI IN MEDICATION ADHERENCE
Using AI in Medication Adherence for predictive insights, adherence risk stratification and personalized support
CONTACT US FOR POCKey Figures from our use case deployment
35-40% NON-ADHERENCE RISK REDUCTION
Reduction in risk of non-adherence from an initial risk of 96%, leading to better health outcome, and fewer hospitalizations
37% IMPROVAL IN INTERVENTION EFFECTIVENESS
Increase in effectiveness of targeted interventions due to accurate identification of specific drivers of non-adherence
IMPROVING OVERALL HEALTHCARE EFFICIENCY
Reducing time/effort spent by healthcare providers on monitoring patient adherence, allowing for more focus on essential tasks
The Need for an AI based Medication Adherence Model
Medication non-adherence is a significant concern, affecting about 50% of patients. This leads to negative health outcome and incurs around $290 billion in avoidable healthcare costs annually. Various factors contributes to medication non-adherence including lack of health literacy among patients, complex multi medication, limited access to care, cost among others. Traditional adherence monitoring measures including patient self-reporting, pharmacy refill data have been ineffective in accurately measuring and improving non-adherence. It is important to identify the top causes of non-adherence per patient and apply custom interventions at the root cause, and this can be an overtly complex process involving volumes of patient data, refill data, prescription claims data, pill counts and other components.
Also, the intervention measures have to be at multiple levels, not just the patients, but also at the provider levels.
This underscores the need of an effective AI based Medication Adherence model leveraging advanced machine learning to measure adherence levels per patient, accurate risk stratification of patients, identify key drivers of non-adherence and recommend intelligent recommendations and interventions per patient to improve adherence.
AI assisted medication adherence has the potential to overhaul adherence monitoring, enhancing patient outcome and improve overall healthcare system efficiency.
Methods to Incorporate AI in Medication Adherence
Finarb incorporates end-to-end solutions for AI based Medication Adherence, utilizing advanced tech tools, data-driven insights, and targeted interventions. Robust Patient PDC (Proportion of Days) Predictive models are constructed using ML algorithms and NLP techniques specifically designed to accurately forecast patient adherence patterns. Performance enhancement of these models is achieved via data augmentation techniques and feature engineering tasks, enhancing the predictive accuracy of the model.
Data analysis is conducted on structured and unstructured datasets to identify drivers influencing medication adherence. Patient risk segmentation forms a critical part of the approach, enabling the design of personalized interventions based on the degree and driver of non-adherence risk. The models undergo continuous refinement, assuring accurate predictions and optimization of adherence strategies. Finarb also integrates intuitive patient follow-ups, custom intervention recommendations, refill reminder systems and various patient empowerment modules through gamification and incentives to enhance the efficacy of AI-based medication adherence.
Finarb makes use of NLP with BERT and Transformer Models, and Machine Learning algorithms like XGBoost and Random Forest as the foundation of our Medication Adherence models. Our models are built to run on cloud-based platforms such as Google Cloud Platform (GCP) and BigQuery. Data pipeline management is handled through Azure Dataflow (ADF), while data visualization is conducted with PowerBI.
Benefits of AI based Medication Adherence
- Achieve optimized non-adherence of 60% post model deployment, from an initial risk of 96%, an almost 35-40% reduction in risk
- Enhance effectiveness of targeted interventions by 30-37%, optimizing resource allocation and reducing wastage.
- Increase patient adherence, improving health outcome, reducing complications, and fewer hospitalizations.
- Save time for healthcare providers by reducing the effort spent on monitoring patient adherence, allowing for more focus on essential tasks.
SCHEDULE A MEETING FOR PoC
If you would like to know more or discuss our use cases in detail